Financial Time Series Generation with GANs
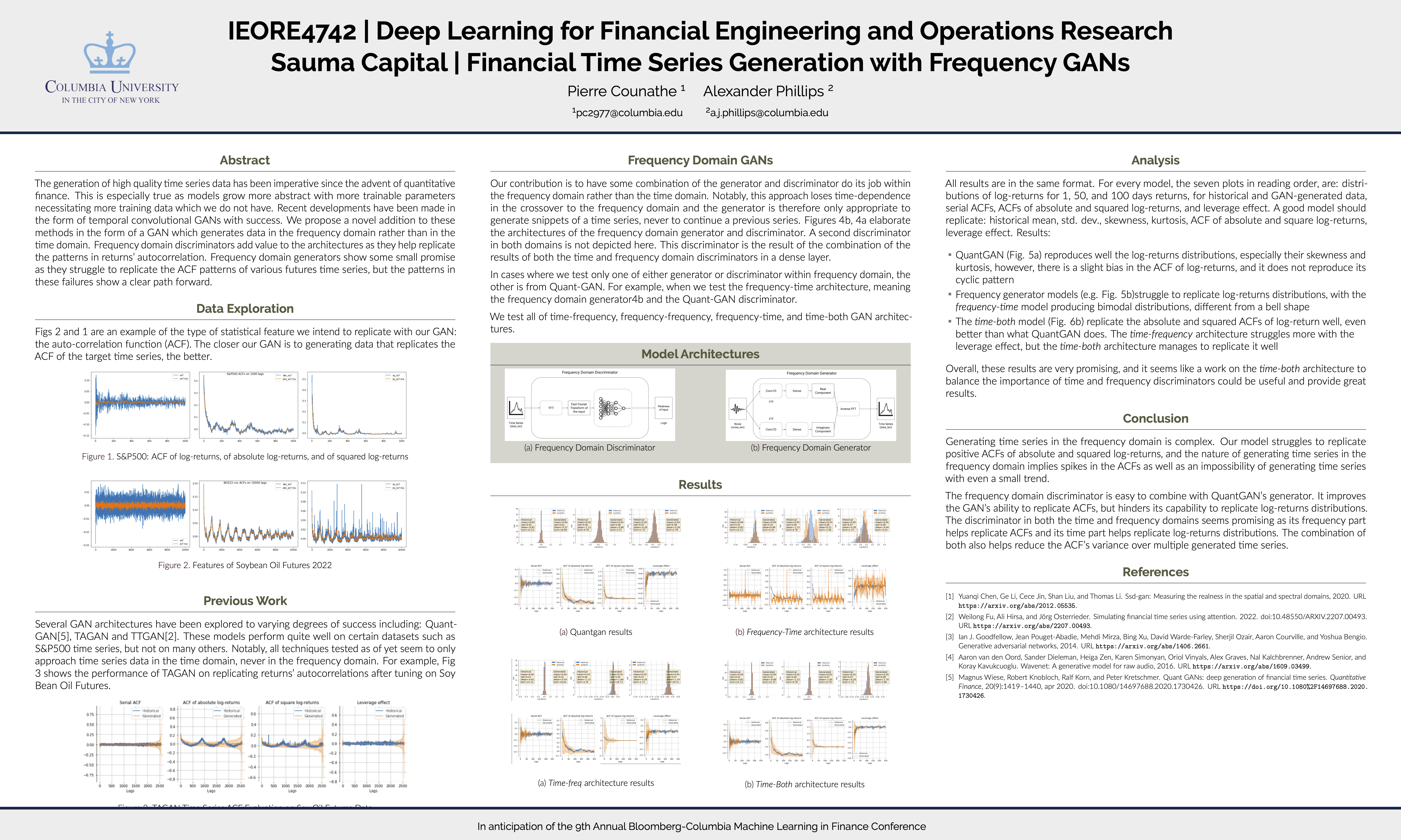
Motivation & Objective
The generation of high quality time series data has been imperative since the advent of quantitative finance. This is especially true as models grow more abstract with more trainable parameters necessitating more training data which we do not have. Recent developments have been made in the form of temporal convolutional GANs with success, notably with the introduction of QuantGAN, TAGAN and TTGAN. We propose a novel addition to these methods in the form of a GAN that acts in the frequency domain rather than the time domain. Frequency domain discriminators add value to the architectures as they help replicate the patterns in returns autocorrelation. Frequency domain generators show some small promise as they struggle to replicate the ACF patterns of various futures time series, but the patterns in these failures show a clear path forward.
Results
See the self-sufficient full-size poster for more details and results.